Note
Go to the end to download the full example code or to run this example in your browser via Binder
“Deconvolution Example”#
This example loads blood DNA Methylation data from GEO, estimates the proportion of cell types, and compares them to known cell type proportions.
Load GEO blood methylation dataset measured on EPIC platform#
from biolearn.data_library import DataLibrary
epic_facs_salas_18 = DataLibrary().get("GSE112618").load()
Estimate cell proportions using EPIC deconvolution model#
from biolearn.model_gallery import ModelGallery
gallery = ModelGallery()
epic_facs_cell_prop = gallery.get("DeconvoluteBloodEPIC").predict(epic_facs_salas_18)
Compare to known cell proportions measured with FACS (fluorescence-activated cell sorting)#
import seaborn as sns
import matplotlib.pyplot as plt
sns.set_style("ticks")
plt.figure(figsize = (5, 5))
sns.scatterplot(x = epic_facs_salas_18.metadata.neutrophils_proportion.astype(float), y = epic_facs_cell_prop.loc["neutrophil"])
sns.scatterplot(x = epic_facs_salas_18.metadata.monocytes_proportion.astype(float), y = epic_facs_cell_prop.loc["monocyte"])
sns.scatterplot(x = epic_facs_salas_18.metadata.nk_proportion.astype(float), y = epic_facs_cell_prop.loc["nk_cell"])
sns.scatterplot(x = epic_facs_salas_18.metadata.bcell_proportion.astype(float), y = epic_facs_cell_prop.loc["b_cell"])
sns.scatterplot(x = epic_facs_salas_18.metadata.cd4t_proportion.astype(float), y = epic_facs_cell_prop.loc["cd4_t_cell"])
sns.scatterplot(x = epic_facs_salas_18.metadata.cd8t_proportion.astype(float), y = epic_facs_cell_prop.loc["cd8_t_cell"])
plt.legend(["Neutrophils", "Monocytes", "NK Cells", "B Cells", "CD4 T Cells", "CD8 T Cells"])
plt.xlabel("Known cell proportion")
plt.ylabel("Predicted cell proportion")
plt.title("Deconvolution Validation - FACS")
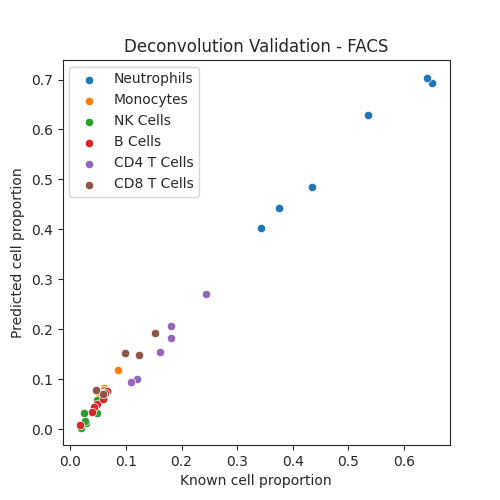
Text(0.5, 1.0, 'Deconvolution Validation - FACS')
Total running time of the script: (0 minutes 0.934 seconds)
Estimated memory usage: 10 MB